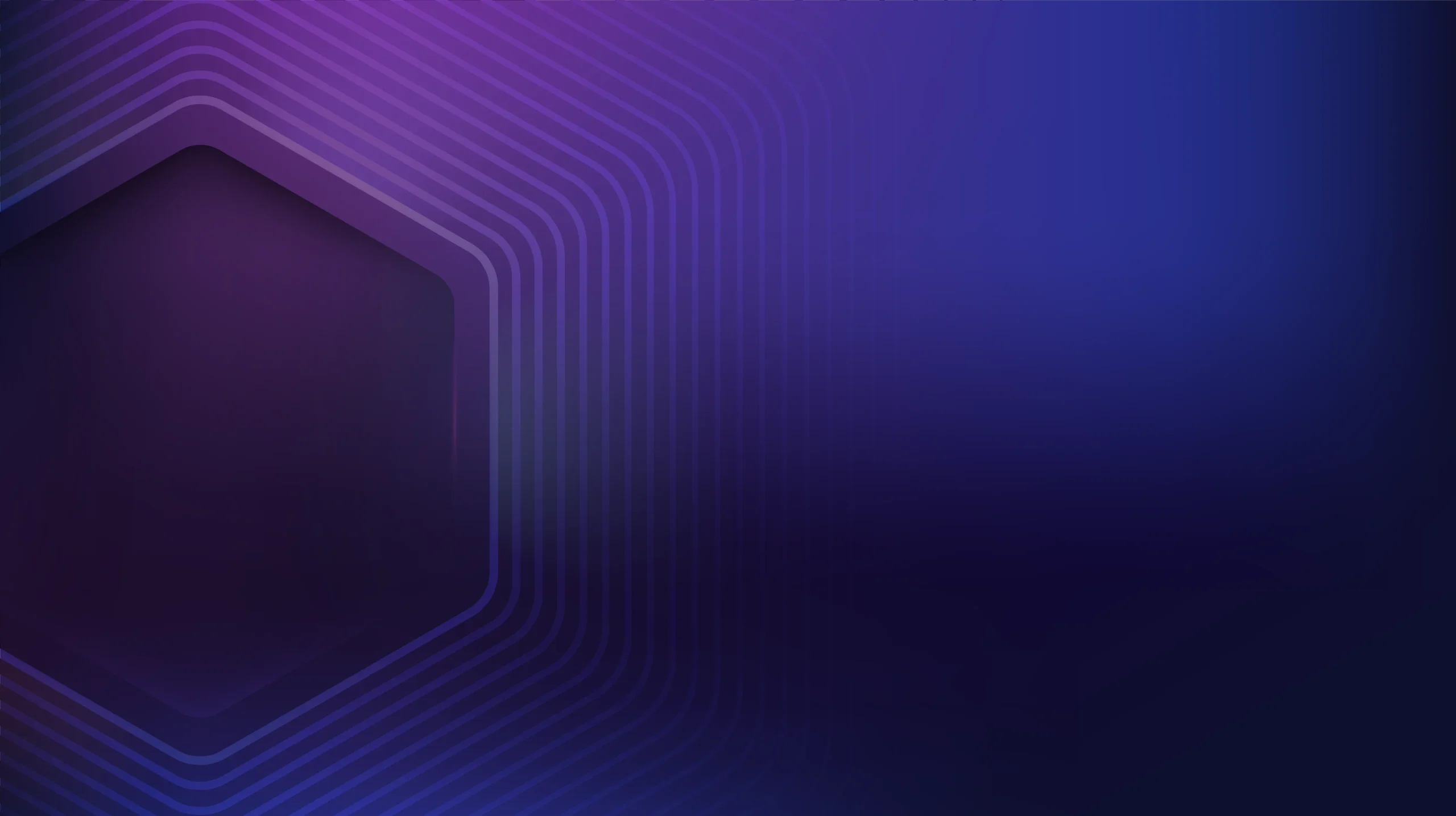
SecurityScorecard Blog
Read the latest blog posts published weekly.
-
Blog
Scorecarder Spotlight: Isabella Dorado Burbano
July 1, 2025Our “Scorecarder Learning & Development Spotlight” series showcases our talented, driven employees, the incredible work they do, and their quest to continue their development as lifelong learners.
More DetailsScorecarder Spotlight -
Blog
What is DNSSEC and Why Is It Important?
June 30, 2025Understand how DNSSEC secures the domain name system with cryptographic methods to verify the authenticity of DNS records and boost DNS security.
More Details -
Blog
What is a Whaling Attack in Cybersecurity?
June 30, 2025What is a whaling attack? See how phishing attacks aimed at executives and whaling attacks work, and how cybercriminals steal sensitive information.
More Details -
Blog
What is a Cyber Attack? Types and Preventive Measures
June 30, 2025Learn what is a cyber attack, explore common types like ransomware and phishing, understand financial impacts, and discover prevention strategies.
More Details -
Blog, Learning Center, STRIKE
Unmasking A New China-Linked Covert ORB Network: Inside the LapDogs Campaign
June 23, 2025SecurityScorecard’s STRIKE team uncovered a new China-Nexus ORB Network targeting the United States and Southeast Asia. Read the report to gain an in-depth look at the LapDogs ORB network, its custom malware, and its role in cyberespionage.
More DetailsSTRIKE Team -
Blog, Learning Center
What Are Proactive Ransomware Prevention Strategies for 2025?
June 3, 2025Ransomware threats are evolving fast. This guide explores the most effective prevention strategies for 2025, from zero trust to supply chain resilience.
More DetailsAttack Surface Management, Tech Center -
Blog
Scorecarder Spotlight: Guillermo Garcia Granda
June 2, 2025Our “Scorecarder Learning & Development Spotlight” series showcases our talented, driven employees, the incredible work they do, and their quest to continue their development as lifelong learners.
More DetailsScorecarder Spotlight -
Blog
Securing the Supply Chain, One API Call at a Time: Inside the SecurityScorecard API Hackathon
June 2, 2025Earlier this month, SecurityScorecard hosted its first-ever API Hackathon, bringing together developers, cybersecurity professionals, and third-party risk managers from around the world to solve real-world security challenges, one API call at a time.
More Details -
Blog, Learning Center
Choosing Your Code Repository: Navigating the Security Landscape of Bitbucket vs GitHub
May 26, 2025Which code repository is more secure for enterprises—GitHub or Bitbucket? Compare their security features, risks, and third-party controls in 2025 to choose the right platform.
More DetailsTech Center -
Blog, Learning Center
What is a Third-Party Vendor? Tips for Managing Vendor Risk
May 13, 2025Third-party vendors play a critical role in cybersecurity exposure. Learn how to define, classify, and manage third-party relationships effectively.
More DetailsAttack Surface Management, Tech Center -
Blog, Learning Center
What is the CIA Triad? Definition, Importance, & Examples
May 12, 2025What is the CIA Triad? Definition, Importance, & Examples to guide an organization’s security procedures and policies. Learn more below!
More DetailsTech Center -
Blog, Learning Center
What Is a Cybersecurity Audit and Why Does it Matter?
May 12, 2025A cybersecurity audit is essential to protecting your organization. Learn key steps, tools, and considerations to perform an effective audit in 2025.
More DetailsTech Center